This study by Guy Carpenter revalidates the findings of the Under the Lens: Investigating Cyber Vendor Model Divergence report, based on the latest model versions while expanding the scope to include tail losses. Revenue continues to be the key driver of model divergence at the mean and was found to be an even more significant factor at the tail. Industry sector and country of domicile, on the other hand, were less important at the tail, where affected entities are highly correlated and individual characteristics are less distinct. Impact of coverage on model divergence varies and is liable to fluctuate based on vendors’ reaction to recent events and the current threat landscape.
A Changing Vendor Landscape
Cyber catastrophe modeling is constantly in motion, due to ever-evolving threats and a continued drive to advance the precision and capability of the models. Guy Carpenter’s Under the Lens: Investigating Cyber Vendor Model Divergence study examined the observed divergence across 3 leading cyber catastrophe models, CyberCube Portfolio Manager, Guidewire Cyence and Moody’s RMS, using predictive analytics. Since the original study’s publication in June 2023, all 3 vendors have released annual updates to their model methodologies and parameters. (Model versions are v5 for CyberCube, M6 for Guidewire Cyence, and v7 for Moody's RMS.)
As they add more granularity and sophistication—and as new vulnerabilities and attack vectors appear—the models offer differing reactions to each input. Macro views of cyber aggregation potential may also evolve, potentially leading to greater divergence across the models. This study re-examines the variability across the updated models. Additionally, the scope of the earlier study has been expanded to encompass model divergence at tail return periods and incorporation of technographic information. We hope this updated study will foster deeper understanding of the modeling space and encourage the informed creation of modeled views of risk.
Our initial analysis focused on 3 specific areas:
- Input parameters that drive the greatest cyber model divergence.
- Identifying market segments where industry view of risk is most divergent.
- Highlighting risk characteristics for which a given cyber model may yield a significant average annual loss (AAL) penalty.
We conducted the study by running a sample portfolio of risks through each catastrophe model to generate the respective modeled AAL. Divergence was next quantified as the coefficient of variation of the modeled AALs for each risk. We then used a combination of individual risk attributes, consisting of policy and coverage detail, to explain the observed divergence for the sampled cohort of risks.
Although we were able to extract valuable insights using the initial approach, we were left with several paths for renewed focus following the first project iteration, the most prevalent of which are the following:
- Given the updated vendors’ models, which input parameters drive the greatest model expected loss divergence.
- Does the same group of input parameters impact variability in the tail in a way similar to the mean?
Guy Carpenter has identified broad themes in the model updates studied. We saw model focus moving away from the traditional cyber data theft/breach scenarios toward greater granularity around ransomware and cloud outage perils. As the cyber environment evolves, so do the perils of greatest importance. Currently, the frequency of attritional ransomware attacks suggests a focus on this method as a vector for large aggregation events. Additionally, as vendors grapple with the breadth of the unknown in the cyber environment, we are seeing significant revisions of return period events and the tail estimates of the models.
For instance, Guidewire Cyence’s loss curve has reduced considerably over the prior version, as Guidewire Cyence removed some of the most severe events from the event set, with a philosophy of ensuring plausibility of the extreme loss events. CyberCube’s new version, however, exhibited significant increases in tail losses, as CyberCube’s view of impacted companies increased for the largest tail scenarios.
These changes highlight the value in updating the earlier study. First, as perils evolve, so will their parameterizations. We need to understand the drivers of divergence in the new models, given the context of a greater focus on ransomware and cloud, along with a departure from data theft.
Further, cyber catastrophe modeling is intended to quantify unlikely and impactful events affecting the cyber industry. Essentially, we are talking about tail events that have been adjusted significantly across the model vendors in their updates and were not a consideration in the prior study beyond their limited impact on the AAL, which is typically driven by higher frequency events. As a result, this study must emphasize tail losses and future periodic review in order to understand the impacts on divergence of the vendor models’ changing views.
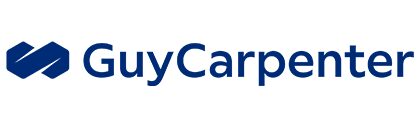
This article is reproduced with the kind permission of ICMIF Supporting Member Guy Carpenter. For more information click here.
Authors: Jess Fung, Shu Iida, Richard McCauley, and Vadim Filimonov.Â
Published May 2024

Key Observations
Our study update demonstrates that the findings from the original study Under the Lens: Quantifying Vendor Model Differences generally hold despite the new vendor model version releases. Key differences driving loss variability across the latest models are detailed below.
The clear top driver of average annual loss variability across the 3 vendor models tested continues to be revenue, as it is closely tied to key cyber loss types, including business interruption (BI), contingent business interruption (CBI) and data restoration. Consistent with the last report, we observed small and micro-sized risks contribute most to divergence. This can be attributed to the lack of granularity in the small and micro risks, especially when it comes to incident, firmographic and technographic data that tend to be less readily available for these entities. As mentioned in the prior report, to allow small and micro risks to be included for modeling, certain assumptions need to be made. Different vendor models rely on different approaches to backfill this missing information, leading to the divergence in model results.
The updated study notes an elevated level of model divergence in the small and micro-risk categories compared with findings in the prior report. This is mainly a result of two vendor models' revised views of small and medium-sized enterprises (SMEs) moving in opposite directions. CyberCube has updated its footprint module based on new technology dependencies data collected, while Guidewire Cyence modeled losses for SMEs decreased considerably from M5 to M6 based on research and market feedback. These changes result in increased volatility across models as measured by the coefficient of variance (CV) for the small and micro risks.
The TVaR models largely follow the same pattern we see with the AAL model. Compared with the AAL, annual revenue has become an even more dominant driver of divergence across models at the tail. The highest-severity events are aggregating across numerous large risks with significant loss potential, thus the risks with higher annual revenue sizes
have greater impact in the tail.
Intuitively, we would expect the vendor models to have a reasonable level of convergence at the high return periods because a fairly contained set of large companies contribute to these losses. However, this is not the case, because the model vendors have diverging perspectives on what could cause the events at the extreme tail and the absolute scale of maximum probable loss. In other words, while it is conceivable that the models agree on large risks driving the tail, the modeled divergence is also the greatest on these revenue bands because the tail is so different.
Industry sector remains a key driver of AAL variability but has decreased from the second-most-impactful characteristic to the third in the refreshed study. Industry sectors differ in how they carry out their business, and in turn, the technologies utilized and relied upon in their work differ. Individual sectors may also vary in their cybersecurity posture, post-incident resiliency, and attractiveness to threat actors. Similar to using revenue, vendor models have applied different treatments and approaches to reflect the nuances between these industry sectors’ characteristics. This naturally translates to higher model divergence, especially as industry sector is an important determinant of modeled results.
Each vendor model has made a conscious and independent decision on the level of granularity for parameterizing their model framework, especially along the dimension of industry sector classification, leading to additional model variability. The latest empirical data shows healthcare to be a top target for cyber-attacks. Based on the divergence observed in the healthcare sector in the latest versions of the 3 models, vendors are likely factoring this change in the threat landscape into their model parameters and scenario narratives differently.

However, as we look to worst-case scenarios where cyber catastrophe events become more widespread, any distinction in individual companies’ industry sector becomes less important because affected entities are highly correlated at that point. Specific industries are prominent drivers of loss at the mean but not the tail. Recent empirical data has shown more cyber loss events on entities within certain industry sectors, but this is a frequency issue that impacts the mean. When we switch focus to the tail scenarios that are predominantly influenced by high severity events, industry sector
becomes less of a driver of loss.
The second-most-impactful driver of model divergence has become country of domicile. All 3 vendor models use US-domiciled exposure as the foundation to construct and parameterize their baseline model, which is a logical and sensible choice given that historically, cyber-related data within the US has been more robust and credible. However, when each vendor extrapolates this baseline to encompass other countries in their expansion of the model coverage area, they employ different methodologies that introduce divergence in model results across countries outside the US.
Secondarily, US risks have the most complete and granular level of cybersecurity posture and risk assessment data available. By taking such data into account, vendor models can produce results that are more appropriate and meaningful, based on the portfolio’s underlying risk quality. The differentiating methodology each vendor model uses to ingest the cybersecurity and risk assessment information leads to US-domiciled risks showing the highest volatility in
modeled AAL.
In looking at the TVaR model, any distinction in individual companies’ country of domicile becomes less important because affected entities are highly correlated at the tail. Similar to industry sector, country of domicile becomes less of a driver of loss within tail scenarios that are predominantly influenced by high severity events.
On a coverage level, some cyber coverages are more impactful in driving the model divergence than others. For instance, the provision of ransomware coverage is not among the top drivers of model divergence, even though ransomware is undoubtedly one of the biggest concerns on the minds of cyber industry participants.
All model vendors put considerable attention into ransomware and have heightened their focus on this risk in recognition of the recent shift in the threat environment. This change and the empirical evidence supporting ransomware frequency increases have brought the vendor models’ view of ransomware into better alignment. Additionally, consistent with other cyber models, CyberCube now considers ransomware as a separate coverage rather than embedded in the investigation and response coverage, which creates more agreement across models and reduces divergence.
In contrast, data breach coverage represents the fourth highest driver of model volatility. The main reason behind this is that CyberCube is an outlier in its view of loss potential from data theft. Since the prior version, CyberCube considers data-theft scenarios as more impactful in the model loss results than do Guidewire Cyence and RMS. This relativity among the models’ views remains unchanged in the new versions, even after CyberCube reclassified certain data theft scenarios as ransomware scenarios.


Conclusions & look ahead
As empirical data grows and the threat landscape changes, cyber model vendors continually release new versions of catastrophe models. The availability of more data points for the validation and calibration of the latest models is the impetus for this updated report. Our research has shown that the conclusions from the original study still hold, in that revenue and industry sector continue to be the top drivers of divergence across the CyberCube, Guidewire Cyence, and Moody’s RMS cyber catastrophe models. Model divergence of tail losses is consistent with mean losses, and for some
characteristics, divergence is more prominent in the extreme tail. Distinction in coverages also leads to varying degrees of divergence, but to a lesser extent.
In comparing the results between this study and the prior one, we have observed consistency in the divergence among the 3 models, but the modeled losses themselves are not necessarily converging. Ultimately, the 3 model vendors have established their own methodologies and views of cyber risk.
As long as none of them introduces any significant changes to their methodologies or view of risk, we expect the observations of this study will continue to be valid. However, as models evolve and more data becomes available, Guy Carpenter will continue to expand our work of quantitatively examining model results and adding new points of comparison, such as secondary modifiers including security scores and security postures. As cyber catastrophe models
gain more traction and become increasingly relevant for enterprise risk management and rating agency considerations, it will be crucial for all cyber (re)insurance market participants to understand loss estimates generated by the leading cyber catastrophe models.